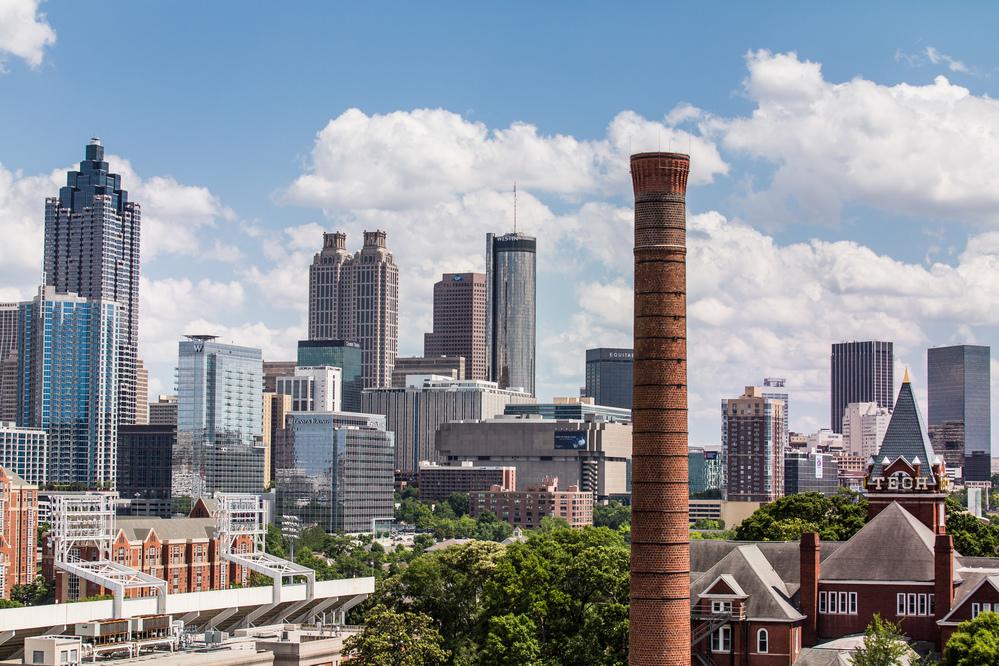
The World Economic Forum Global Agenda Council on the future of universities was absorbed earlier this year into several other councils–a mistake, in my view, since none of the other councils have institutional focus–but several of the white papers live on. This one on the privacy issues inherent in learning analytics generated some interest in 2012, but the big data aspects of higher education seemed like an abstraction to many council members. Over the past year it has started to loom large (see here and here, for example). I happen to be a big fan of analytics. Data from the 700,000 students enrolled in Georgia Tech’s Coursera MOOCs have already had an impact on the quality of residential instruction. However, one of my day jobs is cybersecurity, which has made me sensitive to new technologies that have not paid sufficient attention to security and privacy. This white paper is a note of caution.
The Context
One of the advantages of MOOCs and other recent advances in online education is the potential for analyzing large amounts of fine-grained student data to personalize and customize educational experiences, curriculum, and learning outcomes. The promise of this kind of analysis – called Learning Analytics – for dramatically improving learning at reduced cost is enormous.
These new technologies are, however, not without risks. This working paper discusses one such risk: the threats to individual privacy simultaneously posed by technology and the propensity of large institutions (government, academic, and corporate) and smaller “bad actors” to conduct surveillance on individuals. These threats are substantial and could undermine the development and deployment of advanced technologies.
At least one company has started to develop interfaces with learning management systems, online platforms and academic back office systems. Others have engaged in public discussion of similar plans, and more traditional technology suppliers have clear commercial interests in the development of learning analytics tools based on so-called “Big Data” approaches that aggregate massive amounts of sharable, individualized learning activities in a data analysis pipeline.
The sharability of student data is a key element of learning analytics because the mathematical approaches that can be usefully applied become more powerful when many analytic tools can be brought to bear on data warehouses that have well-defined, open interfaces. In effect, learning analytics enables global sharing of private data. Sharing at this scale may involve storing student data “in the cloud” but there are also institutional approaches in which hundreds of millions of transactions are stored in local data centers and made available to administrators, professors, academic staff, government agencies, families, and institutional researchers.
Inevitably, individual learning records will be combined with external data sources like location data, social media, financial records and electronic health records. It is currently beyond the state of the art to share such data while simultaneously:
- Limiting disclosure of private data to unauthorized individuals, and
- Ensuring data utility
What are the Threats?
Privacy threats in this kind of environment are still emerging, but even casual observers have no problem identifying major vulnerabilities that would compromise privacy.
- Cloud storage and other data warehousing methods incentivize both the creation of more data and longer retention time for that data. Both trends increasing the opportunities for compromising privacy.
- There are no currently practical approaches to ensuring the accuracy or consistency of stored data, creating a privacy threat from false reporting of data.
- The combination of personal information with large external data sets can be used to create “new facts” that had not been adequately anticipated by system designers.
- There is an asymmetry of information in which individuals might give permission for collection and storage of private data without being aware of exactly how much data is being collected and how it might be used.
- The nature of big data technologies gives an inherent advantage to big institutions which have interests that are frequently not aligned with the interests of individual learners.
- Machine learning and very large scale database technologies amplify advantages and disadvantages.
Specific risks arise from the ability to mine these large data sets for capricious or other uses – insurance, determining credit worthiness, or law enforcement, for example — beyond those imagined by individual students, system architects, or even university administrators operating in good faith. Here is a short list of what private information might be revealed by learning analytics:
- Learning labels affecting suitability for employment (e.g., “slow learner” vs. “quick study”)
- Medical problems
- Prone to failure
- Past associations
- Emotional instability
- Financial history
- Sexual orientation
- Embarrassing behavior of family members
- Political leanings
- Affairs and personal indiscretions
- Government-issued identification numbers (e.g., Social Security Numbers)
- Current and past addresses and phone numbers
- Credit information
- Legal proceedings
Legal, Technological, and Other Safeguards
Laws and regulations are slow to adapt to new technologies. Big data/analytics is moving at breakneck speed, so governance that addresses the specific needs of learning analytics is virtually nonexistent. In fact, laws themselves sometimes pose a more significant risk than technology. In the US for example, much of the legal framework for big data privacy stems from a 20 year old law called the Electronic Communications Privacy Act (ECPA) of 1986. Attempting to shore up protections for stored electronic communications, the ECPA requires court-ordered warrants for unopened email. Gmail did not exist in 1986, so the ECPA – anticipating that large stores of email would be rare — requires the much lower standard of relevance to an investigation for documents stored in the Cloud, opened email, and unopened email more than six months old. The EU has attempted to get out in front of the technology with a Data Protection Directive, but it is already out of date.
Other legal safeguards (e.g, In the US FERPA, the Federal Educational Rights and Privacy Act of 1974) literally predate the computer age. FERPA, when applied to new technology is a cumbersome, costly, and ineffective tool for protecting privacy.
Service providers such as Google have been the last line of defense of privacy rights. Google for example issues a “transparency reports” that document governmental data requests (many of which are denied). Other providers have been less reliable protectors of private information. For example, Yahoo!™ has admitted providing information to repressive governments. This information was used to identify political dissenters.
We have no experience with providers of learning analytics, so we cannot yet rely upon self-enforcement by these organizations to ensure effective enforcement of privacy policy. The number of new entrants alone poses a significant risk.
Technological safeguards are very primitive and rely mainly upon adherence to security standards. Google, Twitter, and other providers default to HTTPS to protect private data. Others require full session encryption. These are necessary and useful methods for data protection, but they are not adequate.
The ability of attackers to get around standards-based approaches is well known. Hackers coordinate attacks from thousands or hundreds of thousands of servers by exploiting weaknesses in CAPTCHA puzzles. Social networking sites have recently begun using “social authentication” as a way of identifying robot attacks, but even if such approaches worked for learning analytics, it would solve only a small piece of a very large problem.
Conclusion
Privacy of student data is a primary concern of traditional colleges and universities. The conversion of student data to electronic records in education has parallels with the conversion to electronic medical records in the health arena. We recognize these challenges, and believe that the risk/reward structure of emerging technologies in education will drive both technology and governance in education in much the same way as in health-related fields. Learning analytics, however, adds the new dimensions of scale and precision to what would have otherwise been a systemic but manageable information management problem.
The privacy risks inherent in the rapidly advancing field of learning analytics are significant and rising. Any solution would have to give end uses fine-grained control over information sharing, but fine-grained control is both complex and costly. Students can easily be overwhelmed with choices. End users, when faced with such complexity, inadvertently rely on privacy settings, often resulting in unintended release of information that they intended to be private.
Experience has shown that adding security and privacy safeguards as an afterthought to technological development is ineffective. We hope that all stakeholders in higher education that this historical opportunity to help ensure that privacy is built into the fabric of new systems and that governance models are redesigned to recognize the new risks that are posed as a result of technological innovation.